You can enhance your insights into customer preferences by using five powerful AI techniques for analyzing feedback and reviews. First, Natural Language Processing (NLP) transforms unstructured data into actionable insights. Second, sentiment analysis helps you gauge overall customer sentiment by classifying feedback as positive, negative, or neutral. Third, topic modeling identifies key themes within reviews, while clustering techniques group similar feedback to spot trends. Finally, data visualization tools turn complex data into easily interpretable graphs. Together, these techniques can considerably streamline your feedback analysis process and boost customer satisfaction. There's more to uncover about each technique ahead!
Key Takeaways
- Natural Language Processing (NLP) transforms unstructured customer feedback into actionable insights through tokenization and sentiment analysis.
- Sentiment Analysis classifies feedback as positive, negative, or neutral, tracking changes to identify trends in customer attitudes.
- Topic Modeling automatically identifies key themes in feedback, informing product development and marketing strategies by clustering similar words and phrases.
- Clustering Techniques group similar reviews to uncover hidden patterns and prioritize common concerns for effective resource allocation.
- Data Visualization Tools create interactive dashboards to present findings clearly, promoting data-driven decision-making and showcasing key metrics.
Natural Language Processing
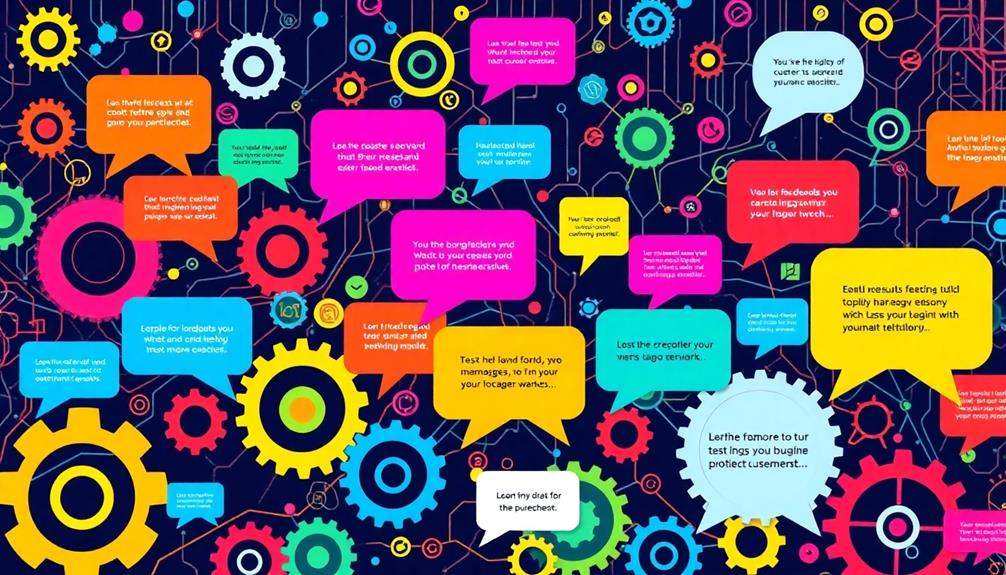
As businesses endeavor to understand their customers better, Natural Language Processing (NLP) plays an essential role in analyzing feedback effectively. By utilizing NLP techniques, you can transform unstructured data from customer feedback into actionable insights.
AI tools powered by machine learning algorithms help break down text through processes like tokenization and stemming, allowing you to extract meaningful themes and intents.
With sentiment analysis, you gain the ability to categorize feedback as positive, negative, or neutral. This categorization is vital for understanding customer sentiments regarding your products or services.
But it's not just about basic classifications; advanced NLP algorithms can perform emotion detection, revealing nuanced feelings like frustration or satisfaction that customers express in their reviews.
Moreover, NLP's capability to analyze text in multiple languages guarantees that you don't miss out on valuable feedback from a global customer base.
By embracing these powerful NLP techniques, you position your business to respond to customer needs more effectively and enhance overall satisfaction.
In the end, harnessing the power of natural language processing enables you to truly listen to your customers and improve their experience.
Sentiment Analysis

Sentiment analysis builds on the foundation laid by natural language processing, taking your understanding of customer feedback to the next level. Using sentiment analysis, you can classify feedback as positive, negative, or neutral, allowing you to gauge overall customer sentiment towards your products or services.
This technique processes vast amounts of unstructured data—like social media comments and online reviews—in mere seconds, enabling you to respond promptly to customer concerns.
By generating sentiment scores and tracking changes over time, you can identify trends and shifts in customer attitudes. This provides critical insights into customer sentiment, assisting in strategic decision-making.
Advanced sentiment analysis tools go beyond simple classifications, employing emotional detection to reveal nuanced feelings within the feedback.
Moreover, continuous learning algorithms enhance the accuracy of sentiment analysis over time. As language use and sentiments evolve, these algorithms guarantee that your analysis remains precise and relevant.
Topic Modeling
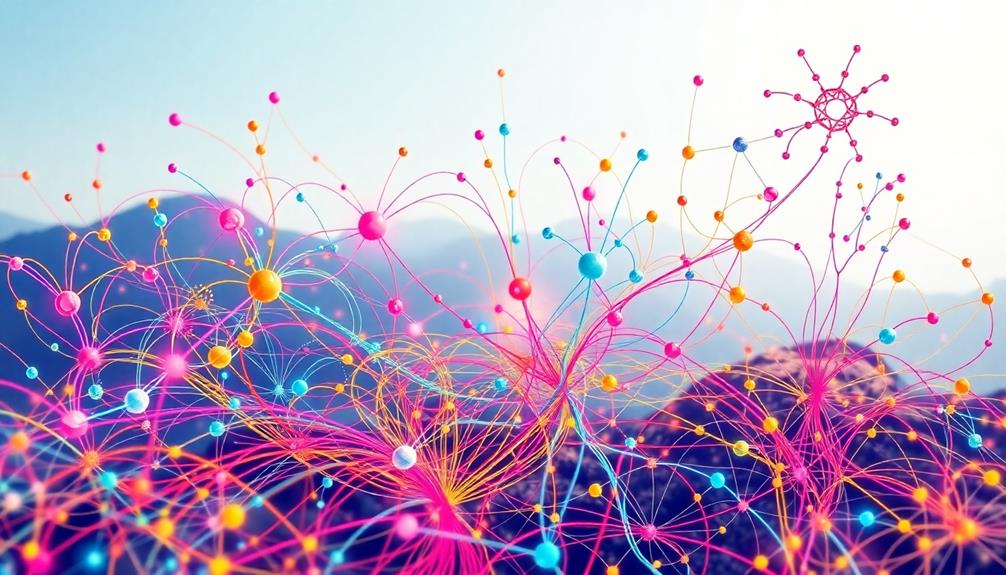
Topic modeling serves as a powerful tool for uncovering the themes hidden within customer feedback. By employing natural language processing techniques, you can automatically identify key topics and gain valuable insights.
Using algorithms like Latent Dirichlet Allocation (LDA), you can cluster similar words and phrases, allowing for effective real-time analysis of vast amounts of data. Additionally, leveraging AI in educational contexts can enhance the analysis process by providing data-driven insights that help in understanding customer sentiment and preferences.
Here are four key benefits of topic modeling:
- Identifying Themes: Understand prevalent themes in customer reviews, such as product features or service issues.
- Spotting Areas for Improvement: Pinpoint specific concerns that need addressing to enhance customer satisfaction and loyalty.
- Guiding Product Enhancements: Leverage insights to inform product development and marketing strategies based on customer feedback.
- Streamlining Analysis: Quickly categorize feedback into relevant topics without needing prior labeling, making your analysis more efficient.
Clustering Techniques
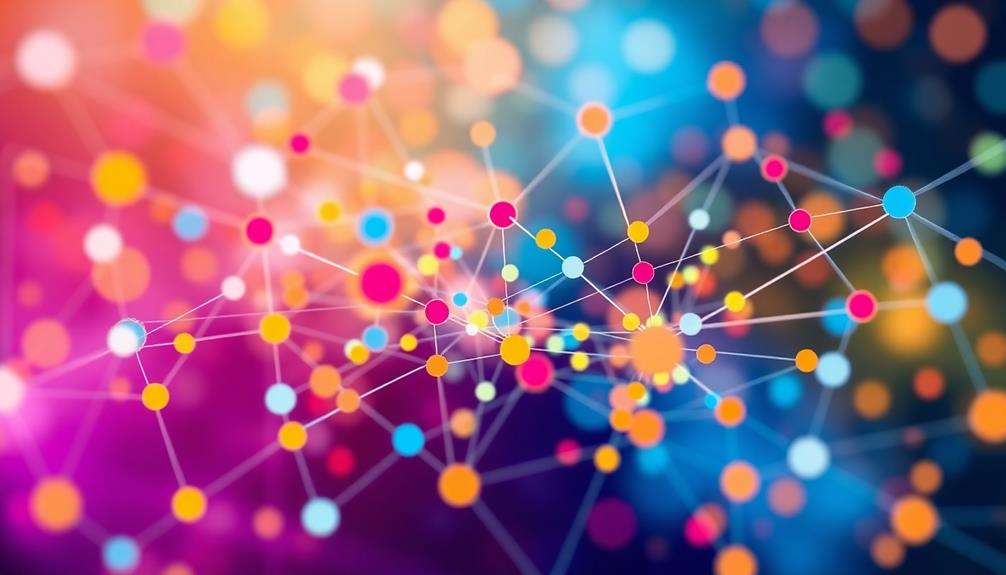
Clustering techniques are essential for analyzing customer feedback effectively. By grouping similar reviews into distinct categories, you can identify trends and common issues within large datasets. Algorithms like K-means, hierarchical clustering, and DBSCAN help categorize feedback based on similarities in sentiment, keywords, or themes, enhancing your understanding of customer perspectives.
Using these techniques, you can uncover hidden patterns in customer feedback that traditional analysis might miss. This allows you to focus on specific areas for improvement. For instance, if multiple reviews cluster around a particular complaint, addressing that concern can considerably enhance customer satisfaction.
Implementing clustering techniques not only provides insights into customer preferences but also boosts operational efficiency. By prioritizing the most frequently mentioned concerns within each cluster, your business can allocate resources more effectively, ensuring that the most pressing issues are resolved first.
Data Visualization Tools
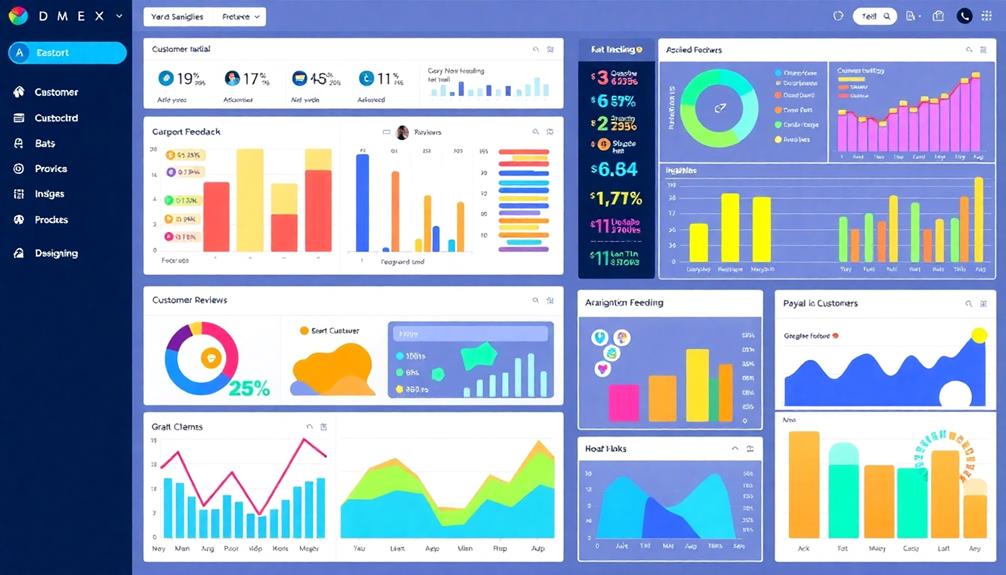
Data visualization tools are game changers when it comes to analyzing customer feedback. They transform complex customer feedback data into intuitive graphical representations, making it easier for you to spot trends and insights quickly.
With the help of popular tools like Tableau, Power BI, and Google Data Studio, you can create interactive dashboards that effectively present your findings. Additionally, these tools can enhance your understanding of customer sentiment by integrating AI techniques for predictive analytics that anticipate customer needs based on feedback trends.
Here are four key benefits they offer:
- Real-Time Updates: Integrate these tools with AI feedback analysis systems for instant visualizations based on the latest customer sentiment data.
- Highlight Key Metrics: Visualizations can showcase overall sentiment scores, topic frequency, and demographic variations, making it easier to identify what matters most.
- Strategic Decision-Making: Use clear visuals to inform stakeholders, guiding your organization's strategic choices effectively.
- Facilitate Data-Driven Discussions: Presenting findings visually promotes engaging conversations around customer feedback, enabling actionable insights.
Frequently Asked Questions
What Is the AI Tool to Analyze Customer Feedback?
To analyze customer feedback, consider using tools like Survicate or Akkio. They automate sentiment categorization, process large data volumes quickly, and help you gain actionable insights to improve your business strategies effectively.
How to Use AI to Analyze Reviews?
You've got a treasure trove of reviews! Immerse yourself, let AI sift through them like a magical sorting hat, categorizing sentiments, spotting trends, and revealing insights that'll make your marketing sparkle and shine.
How to Use AI for Customer Insights?
You can leverage AI for customer insights by analyzing feedback data, identifying trends, and understanding sentiments. This approach helps you refine your strategies, tailor products, and enhance customer experiences based on actionable insights.
Can You Use AI for Feedback?
Imagine a skilled detective piecing together clues. You can use AI for feedback, sifting through data rapidly to reveal trends and sentiments, helping you understand your customers better and address their needs effectively.
Conclusion
Incorporating these AI techniques is like having a compass in a dense forest of customer feedback. Just as a compass guides you to your destination, NLP, sentiment analysis, topic modeling, clustering, and data visualization steer your understanding of customer opinions. By harnessing these tools, you can uncover valuable insights that drive your business forward. So, don't just wade through the feedback; let AI illuminate the path to better customer experiences and informed decisions.