AI-driven sales forecasting leverages advanced tools and techniques to enhance prediction accuracy and optimize strategies. You’ll find methods like time series analysis and regression techniques, which help identify trends and relationships in sales data. Popular tools, such as Aviso and Pipedrive, integrate these methods to provide actionable insights. As you explore further, you’ll uncover how data quality and customer insights play crucial roles in maximizing ROI and refining forecasting approaches.
Key Takeaways
- AI-driven sales forecasting leverages machine learning to analyze real-time data for improved accuracy and reduced bias in predictions.
- Key methods include time series analysis, regression techniques, and ensemble learning to enhance forecasting capabilities.
- Popular tools like Aviso, Pipedrive, and Forecastio provide customizable forecasting solutions and integrate with existing systems for seamless usage.
- High-quality data is crucial; poor data can lead to significant financial losses and inaccurate forecasts.
- Continuous learning and human oversight ensure forecasts adapt to market changes and align with business objectives for optimal performance.
Understanding AI-Driven Sales Forecasting
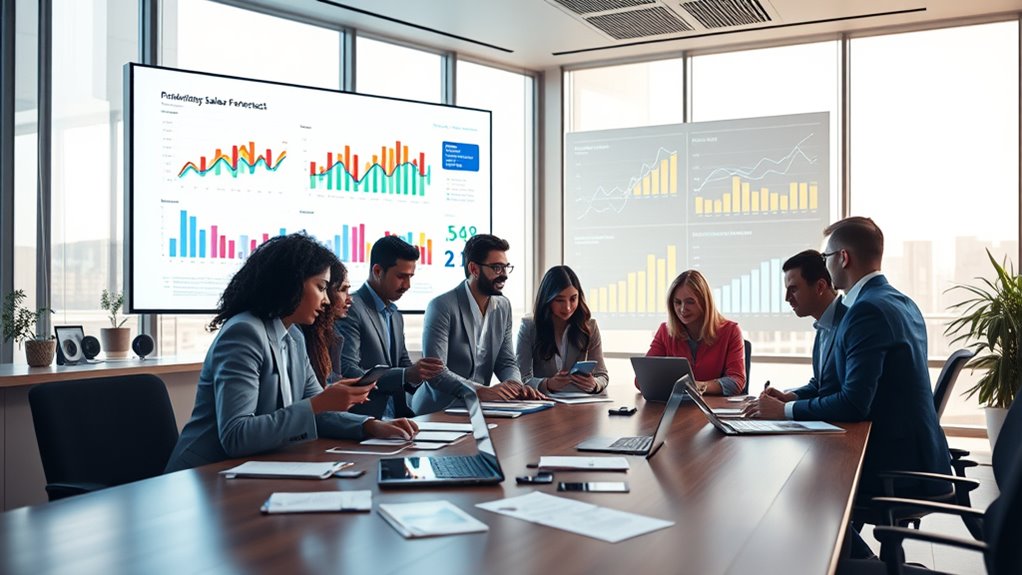
AI-driven sales forecasting is revolutionizing how businesses predict their future sales performance. By leveraging artificial intelligence and machine learning, you can analyze vast amounts of data in real-time, enhancing accuracy and reducing human bias.
This approach delves into customer engagement, deal velocity, communication frequency, product usage, and market conditions to provide deeper insights. As AI models continuously learn and adapt, they refine their predictions, becoming more precise over time.
Rather than replacing human judgment, AI complements it by offering data-driven insights that empower your decision-making. With AI in your forecasting arsenal, you can boost revenue growth, improve efficiency, and better navigate changing market conditions, all while focusing on high-value activities that matter most to your business.
Key Methods for Effective Forecasting
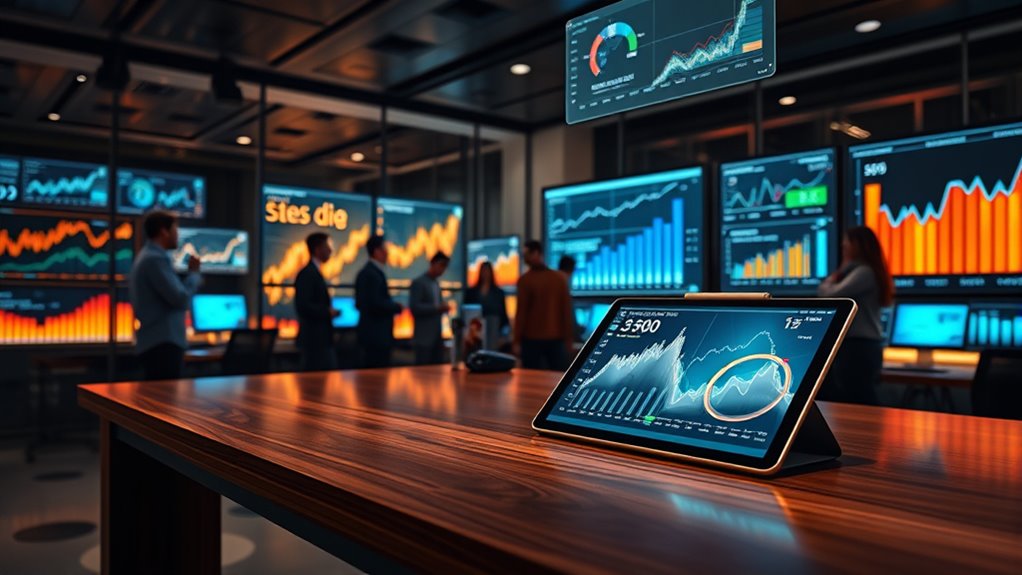
To boost your sales forecasting accuracy, you should consider employing methods like time series analysis, regression techniques, and ensemble learning.
Each of these approaches offers unique insights and can help refine your predictions.
Time Series Analysis
As you dive into time series analysis, you’ll discover a powerful method for forecasting sales by examining data collected over regular intervals. This approach leverages historical sales data—be it daily, weekly, or monthly—to identify patterns and trends.
By recognizing seasonality, trends, and random fluctuations, you can project future sales effectively. Key methods like trend extrapolation and moving averages enable you to smooth out variations and extend past trends into the future.
While time series analysis excels in stable markets, it does have limitations; sudden market shifts or inconsistent data can hinder accuracy. Nevertheless, its predictive power aids in inventory management and resource allocation, making it a valuable tool in your forecasting arsenal.
Regression Techniques
While time series analysis provides valuable insights into sales trends, regression techniques offer a deeper understanding of the relationships between various factors that influence sales performance.
Regression analysis helps you identify how different variables impact your sales, using methods like simple and multiple regression. You’ll need comprehensive historical data for accurate results.
Linear regression models relationships with a simple equation, while non-linear and multivariate regression tackle more complex scenarios. Logistic regression is perfect for predicting binary outcomes, and polynomial regression addresses non-linear relationships.
Ensemble Learning Methods
Building on regression techniques, ensemble learning methods take sales forecasting to the next level by combining multiple models to enhance prediction accuracy. These methods reduce bias and variance, making your forecasts more reliable.
You can use techniques like Bagging, which decreases variance by training on different data subsets, or Boosting, which iteratively refines models to address previous errors.
Stacking combines predictions from various models through a meta-model, while Random Forest leverages multiple decision trees to minimize overfitting.
Advantages of AI in Sales Predictions
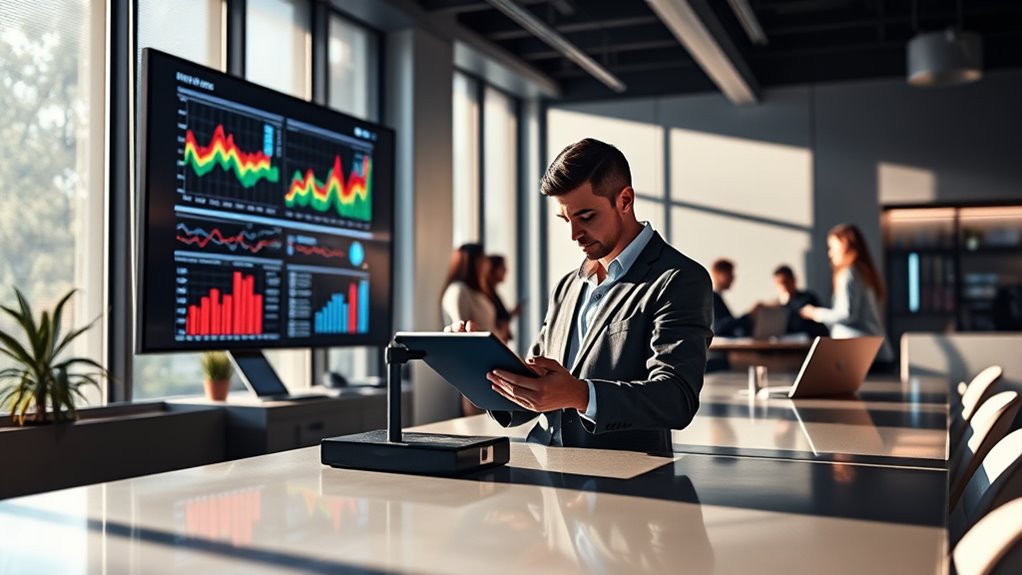
When you leverage AI for sales predictions, you’ll notice a significant boost in forecast accuracy.
By analyzing real-time data, AI helps you make timely adjustments, ensuring your strategies align with current market trends.
This combination not only enhances your decision-making but also positions your business to seize opportunities as they arise.
Improved Forecast Accuracy
AI-driven sales forecasting significantly boosts forecast accuracy by leveraging vast amounts of data to uncover intricate patterns and correlations.
By analyzing both structured and unstructured data, AI algorithms can identify subtle trends that might escape human notice. This data-driven approach eliminates bias, relying solely on insights derived from the data rather than intuition.
As market conditions shift, AI continuously adapts forecasts, ensuring relevance in dynamic environments. With machine learning integration, these models enhance their predictive capabilities over time, learning from past outcomes.
This continuous learning process improves accuracy, helping you make informed decisions. Ultimately, accurate forecasts enable better resource allocation, streamline operations, and support strategic planning, driving your business toward success.
Real-Time Data Analysis
While traditional forecasting methods often struggle to keep pace with rapid market changes, real-time data analysis empowers businesses to stay ahead of the curve.
AI provides instant updates to your forecasts, allowing you to quickly adapt to shifting market conditions and customer needs. By continuously integrating data from diverse sources, AI ensures your forecasts remain relevant and accurate.
This capability not only enhances decision-making through actionable insights but also helps identify potential risks and opportunities early. With AI’s real-time monitoring, you can optimize resource allocation and refine sales strategies based on current trends.
Ultimately, real-time data analysis gives you a competitive edge, enabling proactive adjustments and improved operational efficiency.
Popular Tools for AI-Driven Forecasting
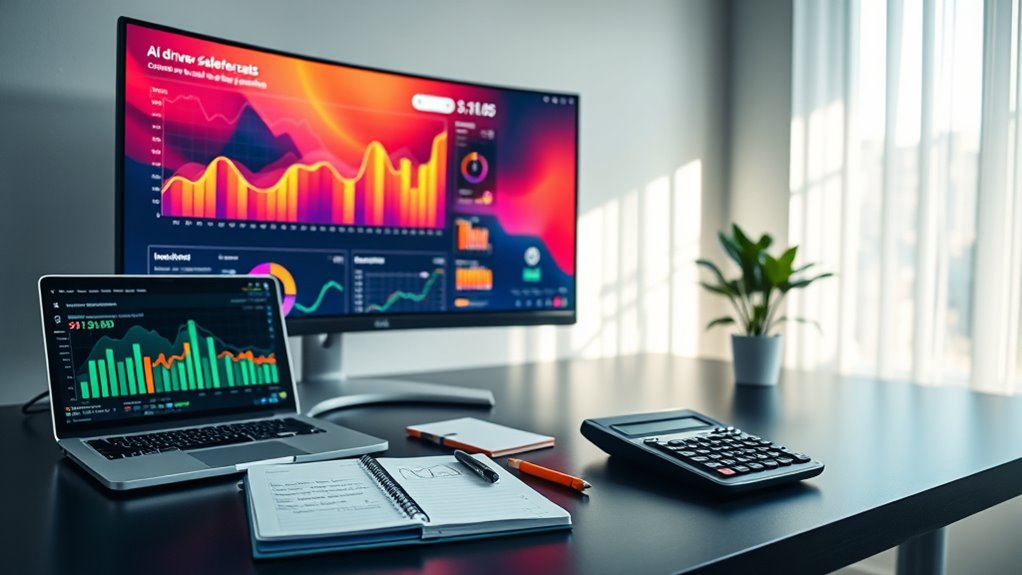
In today’s competitive landscape, choosing the right tools for AI-driven sales forecasting can significantly boost your business’s accuracy and efficiency.
Tools like Aviso focus on deal-level forecasting and personalized coaching, while Pipedrive offers customizable views and predictive analytics.
Avercast utilizes over 200 algorithms for demand planning, and Mediafly excels in hyper-specific reporting and trend analysis.
For those using HubSpot, Forecastio integrates seamlessly for multi-method forecasting.
Each of these tools brings unique features, such as predictive sales analytics in Pipedrive and real-time insights in Forecastio, helping you respond swiftly to market changes.
Evaluate your needs and consider the pricing models to find the best fit for your sales forecasting strategy.
Best Practices for Implementing AI Solutions
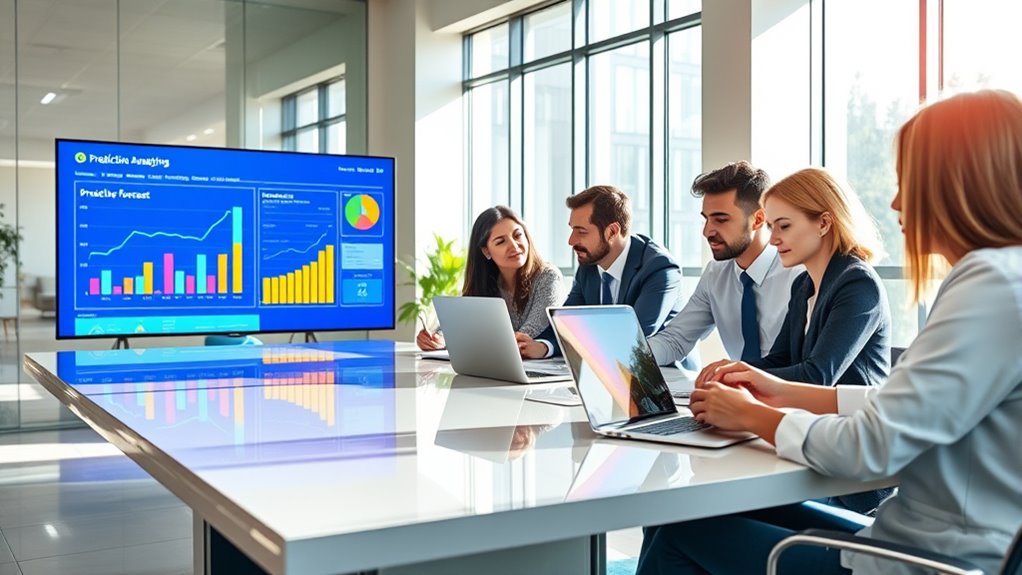
To successfully implement AI solutions in sales forecasting, you must first assess your current processes and identify areas ripe for improvement.
Start by gathering comprehensive historical sales data and ensure its quality through cleaning and integration from various sources. Align your AI objectives with broader business goals to enhance forecast accuracy and optimize resources.
Choose AI tools that easily integrate with your existing systems, are scalable, and user-friendly. Train your AI models with accurate data, and don’t forget to establish human oversight to validate predictions.
Regular updates and performance tracking are crucial for maintaining accuracy. Finally, create feedback mechanisms to continuously refine your approach and adapt your sales strategies based on AI insights.
Assessing the Accuracy of AI Forecasts
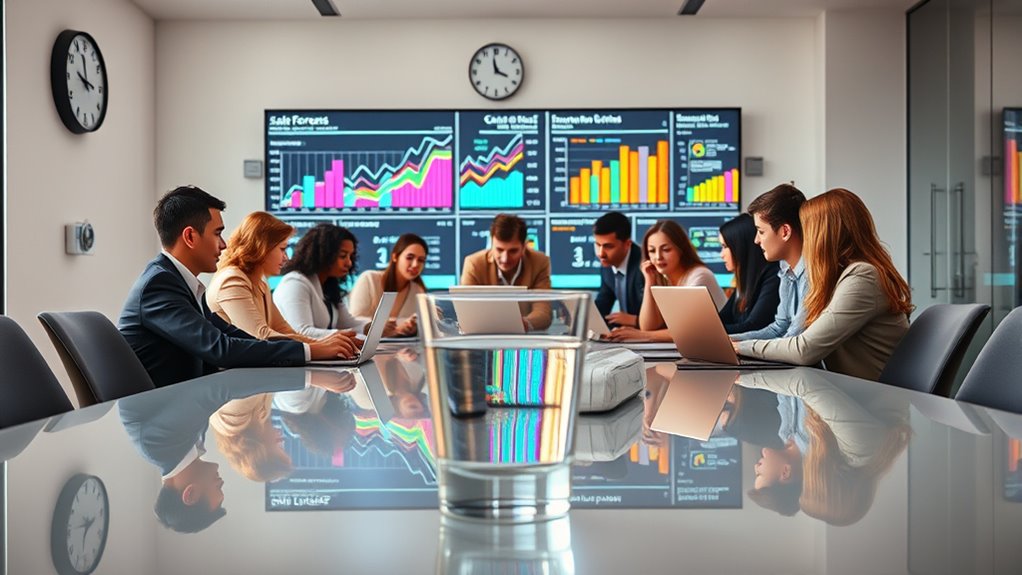
Assessing the accuracy of AI forecasts is essential for ensuring reliable sales predictions that drive business success. You can use metrics like Mean Absolute Percentage Error (MAPE) and Root Mean Squared Percentage Error (RMSPE) to evaluate how closely your forecasts match actual outcomes.
Regular data validation against real sales data helps identify discrepancies, enhancing model performance. Continuous monitoring is crucial, as market conditions change, which may require retraining your AI models to address performance drift.
Selecting the right AI model and fine-tuning parameters based on insights can significantly improve accuracy. Lastly, integrating AI with CRM systems ensures you capture real-time data, fostering adaptability and informed decision-making in your sales strategy.
The Role of Data Quality in Forecasting
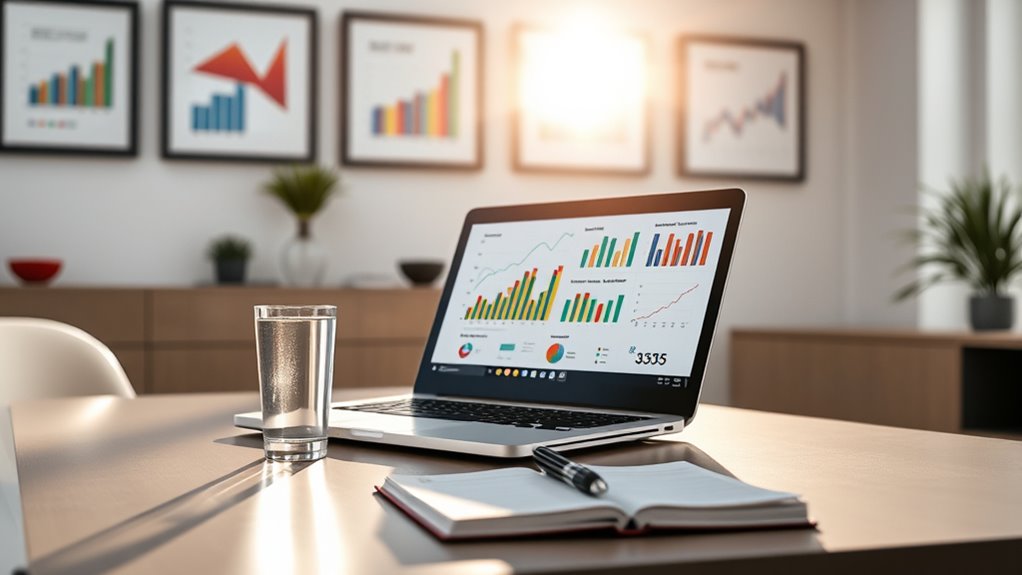
While accurate sales forecasting can drive business success, the role of data quality is often underestimated. Poor data can cost U.S. businesses around $3.1 trillion annually, leading to inaccurate forecasts, missed quotas, and wasted resources.
When data is incomplete, outdated, or duplicated, it hampers your decision-making and negatively impacts customer relations. High-quality data, on the other hand, enhances sales performance and operational efficiency, giving you a competitive edge.
Regular audits, automation, and data governance can significantly improve data quality. By prioritizing accurate data, you not only boost your forecasting abilities but also enhance customer satisfaction and drive revenue growth.
Enhancing Customer Insights With AI
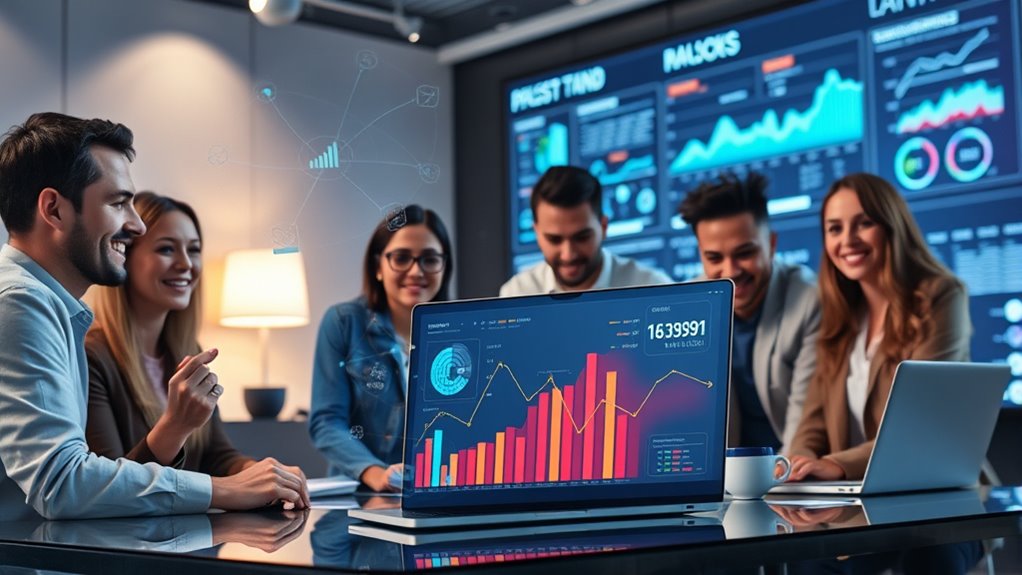
You can significantly boost your understanding of customer behavior by analyzing behavioral patterns with AI.
This approach not only helps you identify trends but also enhances your ability to predict future customer actions.
Analyzing Behavioral Patterns
By leveraging AI to analyze behavioral patterns, businesses can significantly enhance their understanding of customer insights.
AI tools provide real-time data on customer interactions, allowing you to adjust sales strategies proactively. By analyzing engagement patterns, such as communication frequency and response times, you can improve forecast accuracy.
AI identifies subtle behavioral signals that indicate a customer’s likelihood of closing a deal, especially when multiple stakeholders are engaged. Additionally, it adapts forecasts based on shifting market conditions and trends in customer behavior.
These insights enable you to prioritize high-potential deals and optimize your sales pipeline, ensuring you remain responsive to customer needs and market dynamics.
Embracing these AI-driven insights can transform your sales approach.
Predictive Trend Identification
As businesses strive to stay ahead in a competitive landscape, harnessing AI for predictive trend identification becomes essential for enhancing customer insights.
AI algorithms sift through vast datasets, uncovering patterns and trends that sharpen your sales forecasts. By leveraging machine learning models, you can analyze historical data and customer behaviors, allowing for accurate predictions of future sales.
Real-time insights enable you to adjust strategies swiftly based on current market conditions. Additionally, AI tools analyze customer sentiment and behavior, refining your marketing approaches for greater satisfaction. Furthermore, the integration of AI tools for real-time performance tracking allows for continuous optimization of sales strategies based on emerging trends.
Measuring ROI From AI in Sales Forecasting
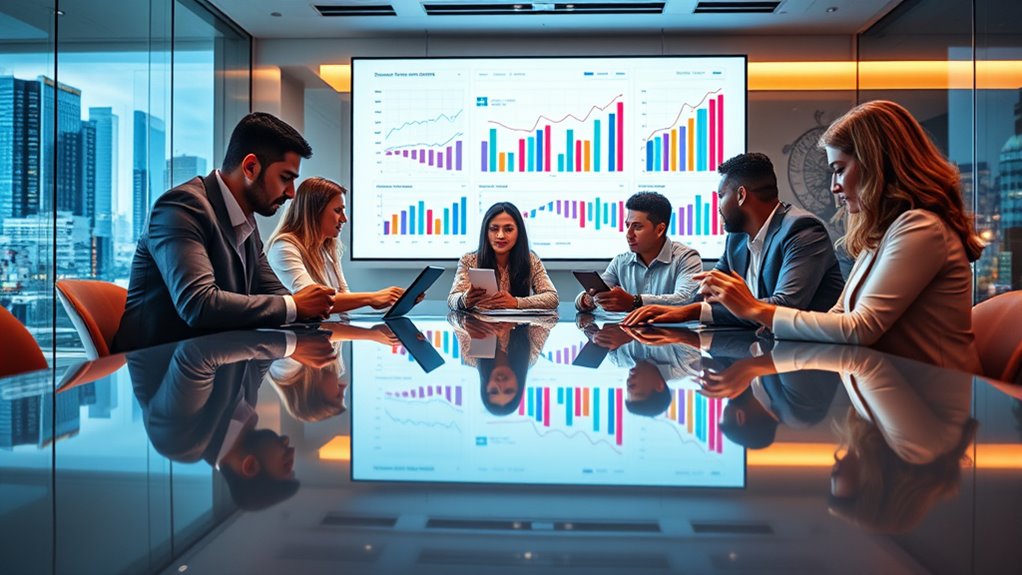
Measuring ROI from AI in sales forecasting reveals significant benefits that can transform your sales strategy. By reducing errors in predictions, AI saves you money on resource allocation and minimizes losses from missed opportunities.
It enhances pipeline management, leading to higher revenue through more accurate forecasts. AI also automates time-consuming tasks, allowing your sales team to focus on high-value activities, boosting productivity.
Key metrics for ROI include tracking forecast accuracy, pipeline velocity, and conversion rates. Implementing AI requires assessing current processes, defining objectives, and quantifying financial benefits.
To maximize ROI, ensure seamless integration with existing systems, prioritize data quality, and continuously monitor performance to refine strategies. Balancing AI insights with human judgment is essential for informed decision-making.
Future Trends in AI-Driven Sales Forecasting
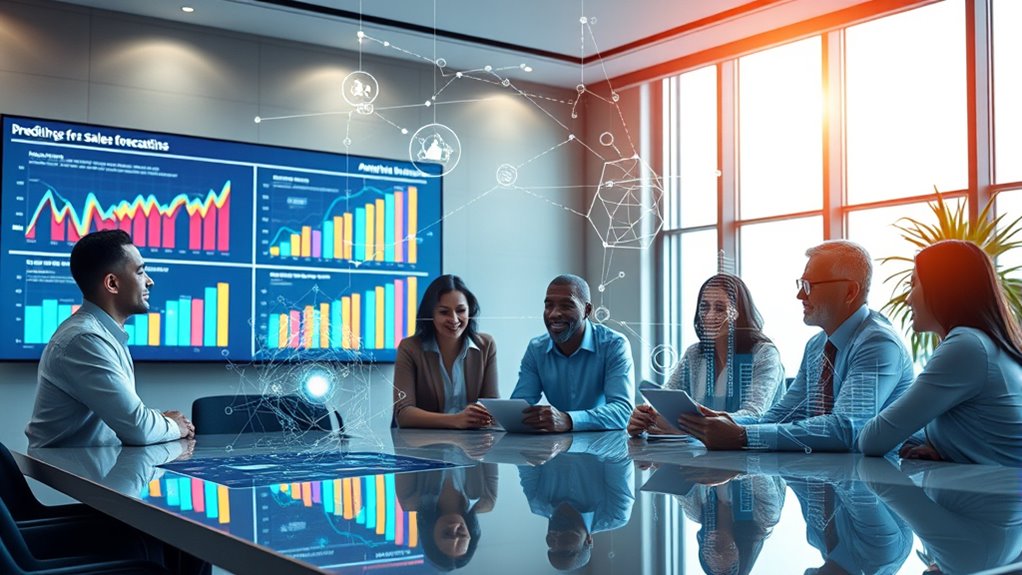
With advancements in technology reshaping the sales landscape, future trends in AI-driven sales forecasting promise to revolutionize the way businesses predict and respond to market dynamics.
By 2025, you can expect accuracy rates to reach up to 95%, significantly outpacing traditional methods. AI’s ability to process vast datasets in seconds means real-time insights for quicker decision-making.
Automation will handle repetitive tasks, allowing your team to focus on strategic planning. Furthermore, AI will continuously learn from new data, adapting to market changes and identifying risks and opportunities.
As B2B adoption rises, leveraging AI tools will provide you with a competitive edge, enhancing sales efficiency and customer satisfaction across various industries, including small businesses.
Frequently Asked Questions
How Long Does It Take to Implement AI in Sales Forecasting?
Implementing AI in sales forecasting usually takes several months to a year.
You’ll start with initial setup, which can take weeks, then move on to integrating data sources, which might require additional time.
Training the AI models can span a few months to ensure they’re accurate.
Finally, full deployment involves a comprehensive integration that may take up to a year, with ongoing updates to keep the system relevant and effective.
What Types of Businesses Benefit Most From Ai-Driven Sales Forecasting?
You’ll find that various types of businesses benefit significantly from AI-driven sales forecasting.
Startups gain accurate predictions for resource allocation, while small and medium-sized businesses can scale effectively to enhance competitiveness.
B2B companies optimize their pipelines, and e-commerce businesses predict demand fluctuations to manage inventory better.
Industries like technology, retail, financial services, and healthcare leverage these insights to improve accuracy, decision-making, and overall efficiency, ultimately gaining a competitive edge in their markets.
Can AI Forecasts Improve Over Time?
Imagine a garden that flourishes with each season; that’s how AI forecasts evolve over time.
As you feed the system more data, it learns and grows, showcasing improved accuracy. With every new leaf of information, it uncovers hidden patterns, much like spotting a rare flower among common blooms.
Continuous updates and error reduction help it adapt, ensuring your forecasts become sharper, making your decision-making process as vibrant and dynamic as the garden itself.
What Skills Are Needed to Manage AI Forecasting Tools?
To manage AI forecasting tools effectively, you’ll need a mix of technical, analytical, and business skills.
Understanding data quality, machine learning, and programming languages like Python is crucial. You should also grasp statistical analysis and data visualization to interpret insights accurately.
Additionally, having strong communication skills and market awareness will help you align forecasts with business strategies.
Lastly, stay updated on AI trends and be ready to adapt your approach as needed.
How Do I Choose the Right AI Model for My Business?
Choosing the right AI model for your business starts with understanding your data volume and the accuracy you need.
Consider your sales environment’s complexity and ensure the model can scale as you grow. Look for options that integrate well with your existing systems.
Evaluate various models like machine learning and regression analysis based on your specific requirements, and always prioritize data quality and relevance to achieve the best results.
Conclusion
Incorporating AI into your sales forecasting isn’t just a trend; it’s a game changer. By leveraging advanced tools and techniques, you can gain deeper insights and make more accurate predictions. Remember, the quality of your data is crucial, so invest time in refining it. As you embrace these innovations, you’ll likely see a significant ROI, ensuring your strategies stay ahead of the curve. The future of sales forecasting is bright—don’t miss out on the opportunity to elevate your approach!